You have decided to participate in a study. You walk into the testing room and in front of you is a cute little snowman — think Olaf from Frozen minus the love for summer — standing beside a computer screen. The robot, named Keepon, is eleven inches tall and fuzzy, and he bounces up and down as he welcomes you to the room.
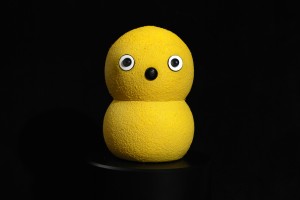
Keepon is a tutor who provides you with tips and hints as you proceed through the study. Run by Professor Brian Scassellati and Ph.D student Daniel Leyzberg at the Yale Social Robotics Lab, the study uses Keepon to investigate the benefits of using a personalized robot tutor on students’ ability to learn. In their recent work, the researchers asked participants to solve a series of puzzles while being coached and helped by the robot. Their findings show that even personalizing the robot’s teaching method in a simple way yields significant benefits for students.
As previous research has shown, there is little debate that learning from a personalized human tutor is much more effective than learning in a classroom setting. Now, researchers are looking to discover whether personalized robotic tutors can be just as effective at teaching. One robot they have developed, known as the Snackbot, uses knowledge of an individual’s history of snack choices to personalize its conversation with the consumer. Another, called iRobiQ, was used as a tutor in classrooms. The main finding common to all of these experiments is that even robots can cater to the individual, and that robotic personalized tutoring is beneficial to students. However, no prior work has specifically isolated the effect of personalization on the way humans interact with robots, especially in an educational setting. And no comparison has been made between personalized tutoring — which consists of tailoring instruction methods to correct a student’s particular mistakes — and individual but non-personalized tutoring.
This is where Keepon comes in. One of the main motivators of this study, as Scassellati put it, is to “find ways to help kids learn difficult tasks.” Scassellati outlined two main questions he hoped the study would answer. “If you received good instruction from a robot,” Scassellati asked, “would that be comparable to good personalized instructions from a person? And can we actually get a robot that has the ability to personalize what it’s doing?”
During the experiment, participants sat in front of a computer and were asked to solve a series of four puzzles. As they did so, they received varying types of help from Keepon. For two experimental groups, Keepon delivered personalized lessons based on an assessment of each student’s skills. These two personalized cases were different in that the order of lessons Keepon delivered was based on two different skill assessment algorithms. For one control group, Keepon delivered lessons but in a completely random order, essentially providing one-on-one tutoring without any personalization. For another group, he simply observed. Keepon was present for every participant in the study regardless of whether he delivered lessons; this ensured that the study compared only the helpfulness of the tutor, and not whether or not participants could see him.
In these experiments, the robot played three roles: host, observer, and teacher. As a host, Keepon welcomed participants and announced the start of the puzzle and when time had run out. As an observer, the robot watched the participant complete the puzzle, his body facing the screen and head following the location of the mouse. As a teacher, the robot delivered tips and strategies to help solve the puzzle three times during the game. When he did so, he would turn to face the participant and bounce his body as he spoke. Visuals appeared on the screen to accompany his teaching tips.
The puzzle that participants worked to solve was a nonogram, chosen because of its relative obscurity to Americans. Participants were given a 10×10 grid with numbers labeling each row and column. The goal of the puzzle was to shade each row and column according to the numbers given. For example, if there was a row marked “4 2”, then within the 10 boxes, participants would shade in a string of four boxes and a string of two boxes, leaving at least one unshaded box in between. Participants had to find a solution that satisfied every row and column.
To assess how much participants had learned over the course of completing the four puzzles, the researchers compared each participant’s completion time for the first puzzle with the completion time for the fourth one. In order to ensure that the difficulty of the first and fourth puzzles remained constant, the researchers gave participants the first puzzle they had completed, rotated by 90 degrees, as their fourth puzzle. In this way, rows became columns, and the difficulty of the puzzles remained constant.
From this experiment, the researchers were able to show that personalized lessons helped players solve three of four puzzles significantly faster than those who had no help or those who had received randomized lessons. The experiment proved that the researchers could produce a robot that personalized its actions, and strongly supported the notion that Keepon’s tutoring helped participants perform significantly better than they could without personalized help. The researchers ran a statistical analysis, which showed that those with personalized lessons improved their performance by one standard deviation above the control. By comparison, previous studies have shown that a human tutor can improve a student’s performance by one to two standard deviations above the mean; this experiment, therefore, suggests that a robot tutor is comparable to a person in giving useful personalized instruction.
The potential uses of this method are wide-ranging, from teaching children difficult topics such as nutrition or second languages to helping autistic children learn social skills. In fact, a group of robots is already working with the Yale Parenting Center to guide adolescents in learning to manage their emotions.
Extra Reading:
M. K. Lee, J. Forlizzi, S. B. Kiesler, P. E. Rybski, J. Antanitis, and S. Savetsila, “Personalization in HRI: a longitudinal field experiment.” 7th ACM/IEEE International Conference on Human-Robot Interaction, pp. 319-326, 2012.
About the Author:Lisa Zheng is a sophomore Molecular, Cellular, Developmental Biology and Economics major in Pierson College.
Acknowledgements: The author would like to thank Professor Brian Scassellati and Daniel Leyzberg for their time and enthusiasm and Julia Rothchild for her help.
Cover Image: Courtesy of Logan Stone