
Earlier this year, Yale Ph.D. candidate Matthew Johnson received a worried e-mail from his advisor. She had just read a paper blasting certain statistical practices in neuroscience research by MIT graduate student Ed Vul. “We’re not doing this, right?” she asked Johnson.
Johnson assured his advisor, Professor of Psychology Marcia Johnson, that her lab was off the hook. None of their projects were examining the relationship between cognitive performance measures and brain activation, the subject of the studies the paper targeted. But Johnson was not surprised by Vul’s claim that many well-known labs, some of which are Yale based, have over-stated the statistical significance of their results. “It’s often the case for laboratory heads to not keep an eye on their graduate students,” he said. “It’s very easy to make these kinds of mistakes.”
Biasing Selections and Inflating Conclusions
The mistake, according to Vul, is “non-independent analysis,” a method of data analysis which potentially inflates results due to a biased data set. If a researcher wants to correlate working memory IQ (WMIQ) with brain activity in a localized region of the brain, a common method is to scan subjects’ brains with an MRI machine as they perform a working memory task, such as reciting a given span of digits backwards.
The researcher then identifies a subset of voxels (three-dimensional data pixels that make up the fMRI scan) within the scan whose activation across subjects correlates strongly with their WMIQ– perhaps he/she will pick voxels exhibiting a raw correlation higher than 0.6 (where 1.0 indicates 100% correlation). The researcher then reports the average of these correlation values as the final correlation between WMIQ and that region’s activity in the brain.
The problem with this method is that the results are based on a biased selection sample. Vul uses the analogy of assessing average investment analyst performance in a company. The performance of each analyst acts as a voxel. An instinctive method would be to identify all analysts whose stock prices reached a certain value and then average those numbers to assess the average performance of the company.
But each analyst, whether talented or not, is affected by luck. Some might have benefited from a particular amount of luck during the period of measurement while others might have been particularly unlucky. Reporting the average of this pre-selected group ignores the fact that some of the numbers are outliers, and, Vul argues, effectively overestimates the performance of the entire company.
Vul began investigating this problem when he noticed many papers giving correlation values higher than .9, which, for neuroscience studies, is wildly improbable. He has shown that by pre-selecting voxels whose activation correlates with the studied behavior, researchers in labs around the country are reporting correlations which are much higher than reality.
Since these correlations are not used for further calculations, no serious problems are immediately apparent. However, Vul notes that statistical correlations can often affect if a paper is published and receives the much-coveted attention of the press.
Stirring up Controversy
After Vul pre-released his paper, which is now published in Perspectives in Psychological Science, on his website at the end of last year, his findings caused quite a splash in the world of neuroscience. A group of scientists, many of whose papers were targeted, have since published a rebuttal, to which Vul published his own rebuttal, and the word is that there’s a rebuttal of the rebuttal of the rebuttal in the works.
Johnson pointed out that Vul’s paper cited many studies done by very prominent researchers in the field. He went down the list of references, identifying the big names. “A lot of these people are pretty distinguished.”
Studies published in collaboration with members of Yale’s MRI group are also listed. In one study, a figure reports a correlation value between neuroticism and activity in the anterior cingulated as 0.8. When asked for comment, the study’s first author, Turhan Canli, Associate Professor of Psychology at Stony Brook University, simply pointed to the rebuttal that has been submitted to Perspectives in Psychological Science by Associate Professor of Psychology Matthew Lieberman of UCLA, author of one of the studies primarily targeted in Vul’s paper.
“We are of course always looking to improve our analysis methods and make sure that the science we conduct is rigorous,” Canli wrote. “In that sense, I support anybody’s efforts to accomplish this goal.”
One key point in Lieberman’s rebuttal was that even if the correlations were overblown, they would still be significant. Going from a correlation of 0.94 to, say, 0.6, still gives a fairly high correlation in the field of science in general. But Vul argued that in other cases, this could result in reporting significant relationships when there are none at all, and Johnson agreed that “it’s a giant problem.”
The real danger, he believes, is when the square of the raw correlation value is reported as too large. Scientists often use r-squared values to show that one factor which highly correlates with another might account for the first.
Therefore, if a scientist reports an r-squared value that is grossly overestimated – for example, 0.94 – the implication is that a certain brain region might account for 94% of cognitive performance in an activity, which does not allow for other regions of the brain to be connected to the behavioral task. If a 66% r-squared value is reported, it leaves the door open for other, uninvestigated regions of the brain to be involved.
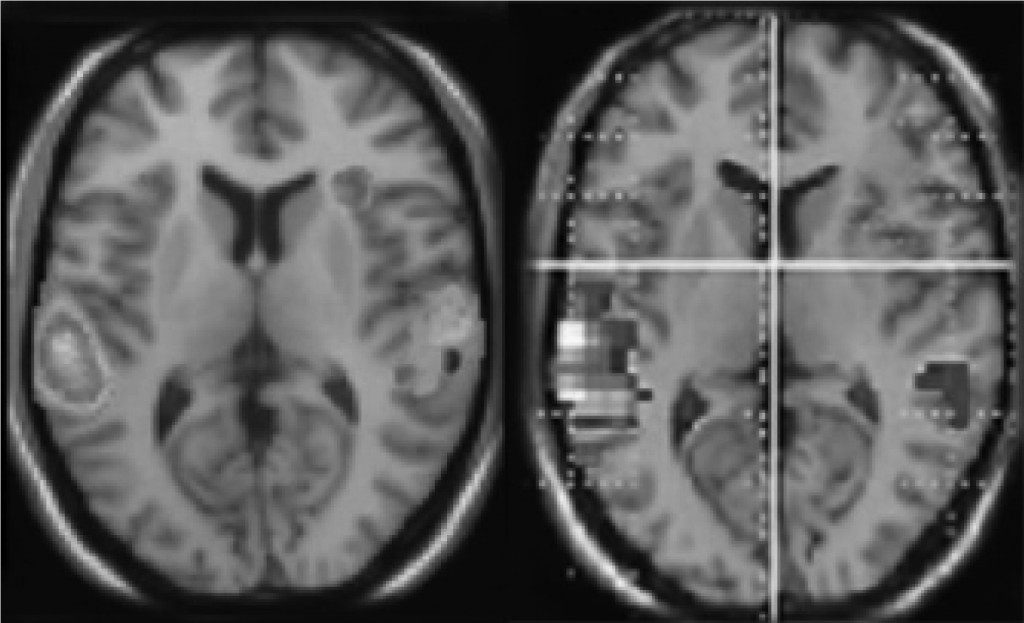
Picking the P-Value
The important thing, says Professor of Psychology Marvin Chun, is to “not be blinded by your own hypothesis.” Chun, a cognitive neuroscientist who focuses on fMRI studies pertaining to visual attention, memory and perception, said that the first thing he looks for in a research paper is the determination of statistical significance.
When you initially look at the data from MRI scans, Johnson explained, you do not see clean areas in the brain light up due to certain behaviors: the whole brain is glowing, because most voxels in your brain are continuously involved in cognitive tasks. There is a host of unconscious cognitive and non-cognitive functions that the body is performing such as heartbeat and respiration.
The key is to isolate the voxels that might be activating as a result, or as a cause, of the studied behavior. The probability has to be determined, therefore, that a particular voxel will show activity due to random chance. This measure is often referred to as the p-value. For example, a p-value of 0.05 means that there is a 5% chance that the activation of a particular voxel is simply due to random chance – meaning that the odds seem pretty good that that portion of the brain may be connected to the studied behavior.
Optimal p-values vary widely across studies: many neuroscientists do not consider 5% to be a stringent enough threshold; others are uncomfortable even with a threshold of 1%. But suppose a researcher adjusts neuroimaging software to display only voxels activated at p-value of .05, and the whole brain is still glowing. Turn the knob down to .01, .001, and it’s still glowing; a p-value can then be reported of .001%.
However, such values are difficult for many to swallow. “These are the hazards of what we call fishing expeditions,” said Professor Frank Keil, another professor in Yale’s psychology department. “You wave your hands and make some kind of post-hoc story. At times I think that can be close to intellectual dishonesty.”
Understanding Data: Useful or Useless?
This leads to a further, even more significant problem. Even when a region of the brain has been shown to correlate with a certain behavior, the information – though expensive to gather – can prove to be almost completely useless. Keil cited research about ADHD as one example of research that sometimes ends up in “a totally empty statement. Every single area of the brain has been hypothesized as being relevant [to ADHD]. It’s incredibly frustrating.”
Keil emphasizes that the value of fMRI studies lies in their ability to contribute ideas of how the brain works and to serve as “a converging form of evidence” in conjunction with studies that do not rely on functional brain scanning. But he urges the public to think of fMRI in terms of a cost-benefit relationship.
The scanners themselves cost on the order of millions of dollars and require hefty service contracts of hundreds of thousands of dollars per year. A typical scan for grant-supported research at Yale’s MRI costs approximately $700, and often the resulting data must be discarded due to the subject coughing or sneezing during the scan. Chun agreed that there were many instances where he deemed that fMRI would not be useful in a study. “It’s just too expensive,” he said.
Partially as a result of the MRI’s cost, many neuroscientists feel pressured to use brain scanning data. “Not personally,” Keil emphasized, as a psychologist who has not used fMRI data in his own research, but “your head gets turned by all the funding initiatives triggered around these.” Chun too felt that there might be some pressure in departments to make sure MRI is used if the resources are available.
Research done by the Keil Lab suggests why. In a study published last year called “The Seductive Allure of Neuroscience Explanations,” Keil and fellow researchers tested the effects of adding irrelevant neuroscience data to “good” and “bad” explanations of psychological phenomena on three subgroups: what they called “naive adults,” students enrolled in an intermediate neuroscience course, and neuroscience experts.
All three subgroups correctly identified the good explanations over the bad ones, but both non-expert groups deemed explanations better with the added neuroscience data, even though it was logically irrelevant to the explanation.
Keil surmised that this might be the reason for added pressure to use fMRI in research about the brain today. “It’s driven by people like congressmen because they fall to the seduction of neuroscience explanations,” he said. “The public is hungry for these data – there’s such a burden.”
Yet fMRI and other forms of brain scanning may still prove useful. Chun feels that any study using fMRI is useful as long as the data collection methods are honest and are conscious of statistical concerns such as those brought to light by Vul. “I’m a bit of an optimist,” he said. “Gung ho, pro-science. Any knowledge is useful knowledge.”
About the Author
NEENA SATIJA is a junior in Branford College, majoring in English.
Acknowledgements
The author would like to thank Professor Frank Keil, Professor Marvin Chun, and Professor Matthew Johnson.