Crops can become contaminated in a variety of ways based on the environments in which they grow. Some examples include deposition from organic chemicals in the air, direct application of pesticides, and ground-water contamination. Image Courtesy of Flickr.
Our food can become contaminated even before it’s pulled out of the ground. As a result of naturally occurring deposition, chemicals applied in agricultural practices can enter crops through the soil and water that plants uptake. Researchers have previously struggled to accurately predict how crops are contaminated due to the complex interactions between crops and their environment. Feng Gao, a postdoctoral associate in the Department of Genetics at the Yale School of Medicine, along with a team of scientists from around the globe, recently used machine learning (ML) to address this problem and shared their findings in the Journal of Hazardous Materials.
Gao tested four existing ML algorithms, programs that formulate predictions for a system based on a sample data set. These four models—Fully Connected Neural Network (FCNN), Gradient Boosting Regression Tree, Random Forest, and Supporting Vector Regression—were utilized to predict root concentration factors (RCFs), the amount of contamination in the root compared to that in the soil around it. Two hundred forty-six data points collected from other studies on crop contamination for eleven crops and fifty-seven chemicals were inputted into the machine algorithms. Gao identified the FCNN as the greatest predictor of RCFs, as indicated by this model’s higher R-squared value and lowest mean absolute error.
This study demonstrates how machine learning, specifically the FCNN, provides a more accurate model for identifying which food is contaminated than did previous approaches. Equipped with this tool, scientists hope to mass-measure and avoid contamination in crop growth, ensuring that our food is safe to eat.
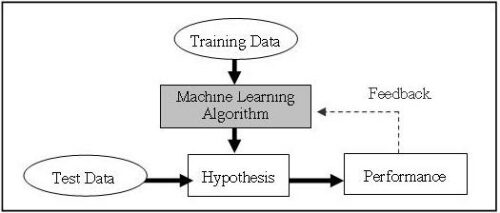