You have just finished watching a great movie on Netflix. However, the night is still young, and you want to watch another movie. But where to start? There are so many different genres…so many different options. Fortunately, Netflix has analyzed your viewing history and immediately recommends a movie catered to your tastes. Your movie experience continues uninterrupted, as you settle in for another “Quirky British Comedy” film.
Such predictive techniques are very common in the fields of finance and social media. Now, according to a recent study conducted by researchers at the Yale School of Medicine, these methods may soon be applicable in the hospital. Yale researcher Dr. Andrew Taylor and his team have developed a series of generalizable analytic methods that can be used to make predictions for patient care. As a proof-of-concept, they focused on sepsis, which is a potentially fatal bacterial infection. As nearly half of all hospital deaths are related to sepsis, the importance of early sepsis prediction is clear.
“The medical field is five or ten years behind other fields as far as being able to make machine-learning based, real-time predictions,” said Dr. Taylor, lead author of the study. “Considering that Facebook tailors ads to users based on previous data, one has to ask why the medical field is not doing something similar to improve patient outcomes.”
In order to predict sepsis risk, physicians commonly use clinical decision rules: a simple scoring system developed through prior studies. Based on score values, different decisions are recommended. For example, if a patient presents with a high heart rate, swelling in one leg, and shortness of breath, clinical decision scores would recommend further testing for the patient. “The problem with this is poor generalizability, because each patient is so unique and these scoring systems are developed in hospital systems with very different patient populations,” said Dr. Kennedy Hall, co-author of the study. “We decided to develop a general approach, rather than a subset of variables, that could be used to predict sepsis and other diseases.”
The specific machine learning based approach used in this study was Random Forest modeling. In this model, random subsets of patient variables are used to create a proverbial “forest of trees.” The trees then essentially vote on whether an outcome—for instance, sepsis—will happen based on the available data. “The beauty of this approach is that, instead of creating a model with four or five variables, we can now use thousands of variables that already exist in the medical health records,” said Dr. Taylor.
Upon comparison with existing prediction models, including several clinical decision rules, Random Forest significantly outperformed the competition. Translated to real numbers, this means that annually, hundreds of additional patients in a hospital can be correctly identified for risk of death from sepsis.
The research team envisions a future where hospitals use analytic methods that are adaptable to their own patient populations. This is a new way of thinking. Instead of developing one specific model, the team proposes that autonomous methods should be developed and shared so that hospitals can learn from their own patients and improve care.
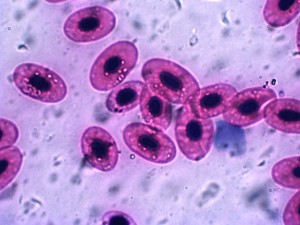
Unfortunately, these predictive techniques have not trickled into the mainstream yet. There is still some reluctance in the medical field to accept the “black box” approach of using machines instead of people. However, Dr. Taylor and Dr. Hall believe that these models should aid, rather than supplant, physician judgment. “We must give up some power to the more versatile and accurate ‘black box’ approach,” said Dr. Hall.
Now having established a proof-of-concept, Dr. Taylor and Dr. Hall are looking for new ways to optimize their approach and extend it to other patient care domains. “The next step is implementation in the hospital,” said Dr. Taylor. “In the next few years, we hope to move from theory to practice and make real differences in patients’ lives.”